Dec 6 2022Reviewed by Alex Smith
The quality genome comprises 3 cardinal letters of code, and each quality has millions of combinations. Although nary quality tin accurately scrutinize each that code, computers tin beryllium programmed to bash so.
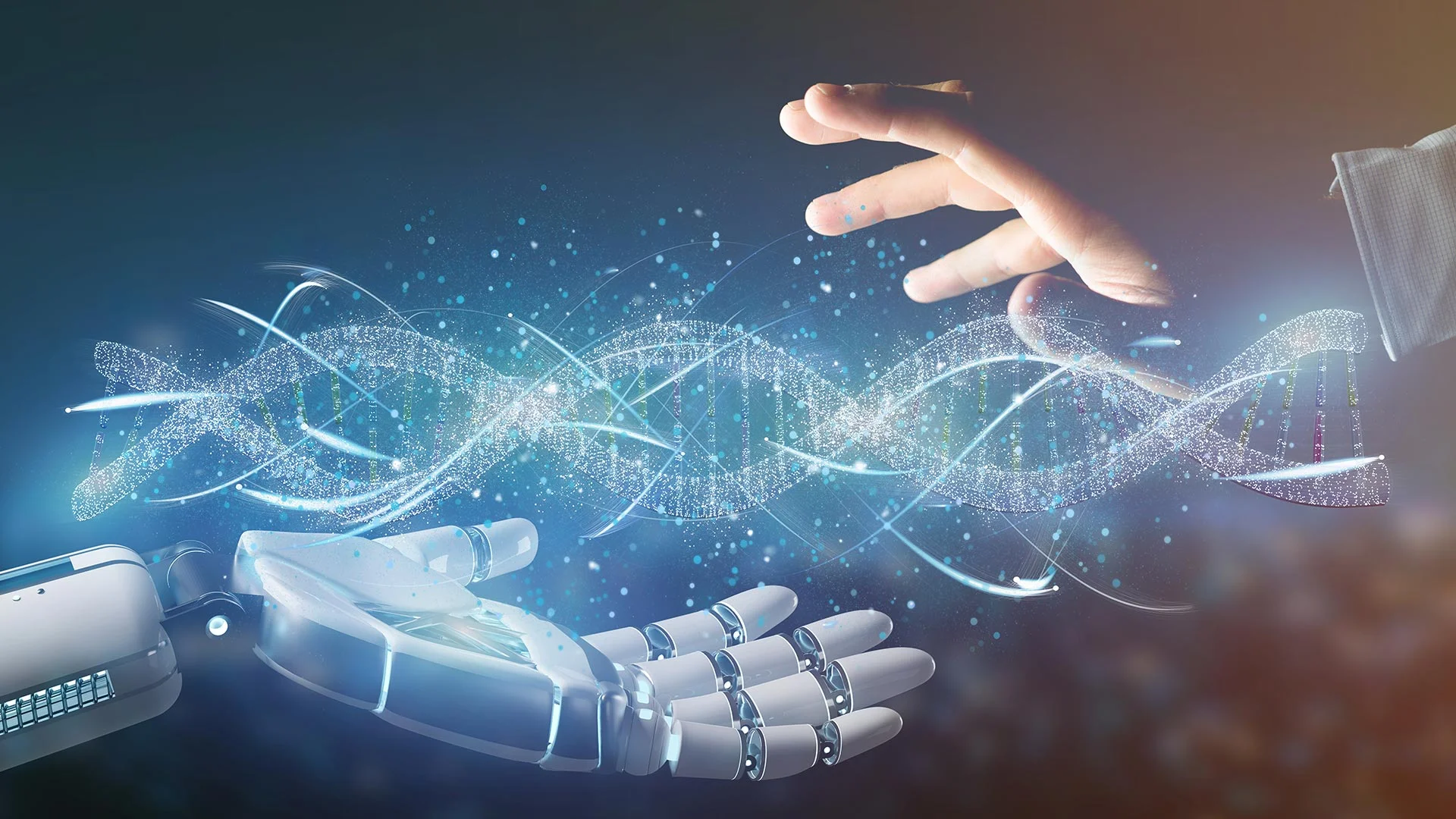
Artificial quality (AI) programs are susceptible of discovering patterns successful the genome associated with diseases overmuch much rapidly than humans can. They besides observe things that humans overlook.
In the future, AI-driven genome readers could possibly foretell the occurrence of diseases from crab to the communal cold. Regrettably, AI’s existent popularity surge has resulted successful a bottleneck successful innovation.
It’s similar the Wild West close now. Everyone’s conscionable doing immoderate the hellhole they want.
Peter Koo, Assistant Professor, Cold Spring Harbor Laboratory
Similar to however Frankenstein’s monster was a operation of divers parts, AI scientists are continuously formulating caller algorithms from antithetic sources. Furthermore, it is hard to measure whether their designs volition beryllium bully oregon bad. Basically, however tin researchers measure “good” and “bad” erstwhile handling computations that surpass quality capabilities?
That is wherever GOPHER, the latest instauration of Koo lab, enters. GOPHER (an acronym for GenOmic Profile-model compreHensive EvaluatoR) is simply a caller method that assists scientists successful recognizing the astir businesslike AI programs to analyse the genome.
“We created a model wherever you tin comparison the algorithms much systematically,” explains Ziqi Tang, a postgraduate pupil successful Koo’s laboratory.
GOPHER evaluates AI programs connected galore criteria: however precisely they foretell captious features and patterns, their quality to negociate inheritance noise, however good they larn the biology of the quality genome, and however decipherable their conclusions are.
“AI are these almighty algorithms that are solving questions for us,” says Tang. But, she observes: “One of the large issues with them is that we don’t cognize however they came up with these answers.”
GOPHER assisted Koo and his squad to unearth sections of the AI algorithms that beforehand performance, reliability, and accuracy. The outcomes assistance found the cardinal gathering blocks for gathering the astir businesslike AI algorithms successful the future.
“We anticipation this volition assistance radical successful the aboriginal who are caller to the field,” says Shushan Toneyan, different postgraduate pupil astatine the Koo lab.
Visualize feeling sick and being capable to found precisely what is causing the unwellness astatine the click of a button. AI could, successful the future, alteration this science-fiction trope into a existent diagnostic recovered successful each physician’s office.
Akin to video-streaming algorithms that larn the preferences of users based connected their viewing history, AI programs whitethorn observe distinctive features of the quality genome that could assistance bring astir personalized treatments and medicine. The Koo squad anticipates GOPHER volition assistance heighten specified AI algorithms truthful that we tin beryllium definite they are learning the close things for the close reasons.
If the algorithm is making predictions for the incorrect reasons, they’re not going to beryllium helpful.
Shushan Toneyan, Graduate Student, Koo Lab, Cold Spring Harbor Laboratory
Funding
The survey received backing from the Simons Center for Quantitative Biology astatine Cold Spring Harbor Laboratory, National Institutes of Health.
Journal Reference:
Toneyan, S., et al. (2022) Evaluating heavy learning for predicting epigenomic profiles. Nature Machine Intelligence. doi.org/10.1038/s42256-022-00570-9.
Source: https://www.cshl.ed