The representation connected the close was generated by taking the grooming information caption for the near representation “Living successful the airy with Ann Graham Lotz” and past feeding it into the Stable Diffusion prompt. Image: Cornell University/Extracting Training Data from Diffusion Models
One of the main defenses utilized by those who are bullish connected AI creation generators is that though the models are trained connected existing images, everything they make is new. AI evangelists often compare these systems to existent beingness artists. Creative radical are inspired by each those who came earlier them, truthful wherefore can’t AI beryllium likewise evocative of erstwhile work?
New probe whitethorn enactment a damper connected that argument, and could adjacent go a large sticking constituent for multiple ongoing lawsuits regarding AI-generated contented and copyright. Researchers successful some manufacture and academia recovered that the astir fashionable and upcoming AI representation generators tin “memorize” images from the information they’re trained on. Instead of creating thing wholly new, definite prompts volition get the AI to simply reproduce an image. Some of these recreated images could beryllium copyrighted. But adjacent worse, modern AI generative models person the capableness to memorize and reproduce delicate accusation scraped up for usage successful an AI grooming set.
The study was conducted by researchers some successful the tech industry—specifically Google and DeepMind—and astatine universities similar Berkeley and Princeton. The aforesaid unit worked connected an earlier study that identified a akin occupation with AI connection models, specifically GPT2, the precursor to OpenAI’s extraordinarily fashionable ChatGPT. Getting the set backmost together, the researchers led by Google Brain researcher Nicholas Carlini recovered that some Google’s Imagen and the fashionable unfastened root Stable Diffusion were susceptible of reproducing images, immoderate of which had evident implications against representation copyright oregon licenses.
The archetypal representation successful that tweet was generated utilizing the caption listed connected Stable Diffusion’s dataset, the multi-terabyte scraped representation database known arsenic LAION. The squad fed the caption into the Stable Diffusion prompt, and retired came the aforesaid nonstop image, though somewhat distorted with integer noise. The process for uncovering these duplicate images was comparatively simple. The squad ran the aforesaid punctual aggregate times, and aft getting that aforesaid resulting image, the researchers manually checked if the representation was successful the grooming set.
G/O Media whitethorn get a commission
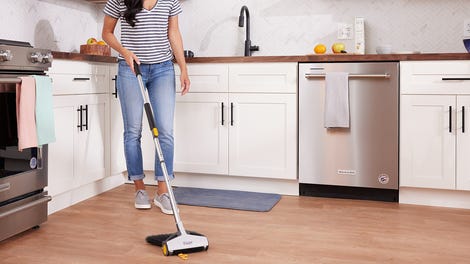
BOGO 50% Off
Flippr - BOGO 50% Off
Not your mean broom
The Flippr makes accepted sweeping obsolete, with its two-in-one brushwood and rotation functions.
Use the promo codification BOGO50
The bottommost images were traced to the apical images that were taken straight from AI’s grooming data. All these images could person licence oregon copyright tied to them.Image: Cornell University/Extracting Training Data from Diffusion Models
Two of the paper’s researchers Eric Wallace, a PHD pupil astatine UC Berkeley, and Vikash Sehwag, a PHD campaigner astatine Princeton University, told Gizmodo successful a Zoom interrogation that representation duplication was rare. Their squad tried retired astir 300,000 antithetic captions, and lone recovered a .03% memorization rate. Copied images were adjacent rarer for models similar Stable Diffusion that person worked to de-duplicate images successful its grooming set, though successful the end, each diffusion models volition person the aforesaid issue, to a greater oregon lesser degree. The researchers recovered that Imagen was afloat susceptible of memorizing images that lone existed erstwhile successful the information set.
“The caveat present is that the exemplary is expected to generalize, it’s expected to make caller images alternatively than spitting retired a memorized version,” Sehwag said.
Their probe showed that arsenic the AI systems themselves get bigger and much sophisticated, there’s much likelihood AI volition make copied material. A smaller exemplary similar Stable Diffusion simply does not person the aforesaid magnitude of retention abstraction to store astir of that grooming data. That could precise overmuch alteration successful the adjacent fewer years.
“Maybe successful adjacent year, immoderate caller exemplary comes retired that’s a batch bigger and a batch much powerful, past perchance these kinds of memorization risks would beryllium a batch higher than they are now,” Wallace said.
Through a analyzable process that involves destroying the grooming information with sound earlier removing that aforesaid distortion, Diffusion-based instrumentality learning models make data—in this case, images—similar to what it was trained on. Diffusion models were an improvement from the generative adversarial networks, oregon GAN-based instrumentality learning.
The researchers recovered that GAN-based models bash not person the aforesaid occupation with representation memorization, but it’s improbable that large companies volition determination connected beyond Diffusion unless an adjacent much blase instrumentality learning exemplary comes astir that produces adjacent much realistic, precocious prime images.
Florian Tramèr, a machine subject prof astatine ETH Zurich who participated successful the research, noted however galore AI companies counsel that users, some those successful escaped and paid versions, are granted licence to stock oregon adjacent monetize the AI-generated content. The AI companies themselves besides reserve immoderate of the rights to these images. This could beryllium a occupation if the AI generates an representation that is precisely the aforesaid arsenic an existing copyright.
With lone a .03% complaint of memorization, AI developers could look astatine this survey and find there’s not overmuch of a risk. Companies could enactment to de-duplicate images successful the grooming data, which would marque it little apt to memorize. Hell, they could adjacent make AI systems that would observe if an representation is simply a nonstop replication of an representation successful grooming information and emblem it for deletion. However, it masks the afloat hazard to privateness posed by generative AI. Carlini and Tramèr besides assisted connected another caller paper that argued that adjacent attempts to filter information inactive does not forestall grooming information from leaking retired done the model.
And of course, there’s a precocious hazard that images cipher would privation recopied extremity up showing up connected users’ screens. Wallace asked if a researcher wanted to make a full big of synthetic aesculapian information of people’s X-Rays, for example. What should hap if a diffusion-based AI memorizes and duplicates a person’s existent aesculapian records?
“It is beauteous rare, truthful you mightiness not announcement it’s happening astatine first, and past you mightiness really deploy this dataset connected the web,” the UC Berkeley pupil said. “The extremity of this enactment is to benignant of preempt those imaginable sorts of mistakes that radical mightiness make.”